Key Takeaways
- Speech enhancement technology often works on either a subtractive or reconstructive basis; our new model, Lark, uses reconstructive technology to repair missing frequencies as well as removing background noise and more.
- Our AI-powered technology does not mimic or replicate someone’s voice; instead, it repairs missing frequencies, avoiding ethical concerns around voice clones and preserving speaker identity.
- Listen to the difference between distorted, subtractive and reconstructive audio samples, and view the differences for yourself in spectrograms.
Our mission at ai|coustics is to make audio accessible for anyone, on any device. Our technology means that no matter how distorted, noisy or low quality the audio you receive or create is, you’ll be able to broadcast a studio-quality and engaging final piece. That’s what makes ai|coustics so beneficial for so many people, from content creators to broadcasters and more. But how do we do it? And how do we make sure that ai|coustics works for every voice, across different accents and languages?
Let’s break down the technology and process behind our speech enhancement. In this article, we’ll be sharing how ai|coustics works, and what makes us a robust, trustworthy and ethical technology to improve every piece of audio.
How does the speech enhancement tool work?
Whether you’re using our API, SDK or the web app, and whether you’re working at a huge scale in a broadcasting context or on individual short-form videos, the underlying technology here at ai|coustics is built on—you guessed it!—AI and machine learning.
There are two typical machine learning models. One follows a subtractive design: as the name implies, this technology is all about removing information from an audio signal. For example, if there’s a lot of background noise, a subtractive machine learning model can help remove the irrelevant information from the signal. So you’ll get the speech of the person you’re interviewing, but not someone coughing or a car going past behind you.
The problem with subtractive models is that sometimes signals get mixed; a subtractive model will struggle to repair broken audio. For example, if you’ve recorded something with a lower quality microphone, or with a microphone that was too far away, you might be missing some higher frequencies of the sound. A subtractive model will be unable to fix those missing frequencies.
That’s why we use another type of machine learning model, often called generative. You’ve probably heard of generative AI, and you might be suspicious of it: it’s the technology that is able, as the name implies, to generate content from nothing. But the way we use generative AI and machine learning at ai|coustics would more accurately be called reconstructive.
What that means is that the model can parse the signal and separate out its threads. For example, it could listen to five seconds of audio and distinguish:
- Your interviewee speaking
- A car horn
- The rumble of traffic
- Someone else talking in the background
- The echo of room resonance
A reconstructive model like ours removes everything except the first and most important signal: your interviewee speaking. And because it’s not just subtractive, if there are points where the signal gets too mixed (i.e. a car horn over your interviewee’s voice), it can use its machine learning database to reconstruct what your interviewee was saying exactly, in their own voice.
Can you show me the difference between a subtractive and reconstructive model?
Sure! Let’s have a listen.
Here’s the original audio sample from a movie, with background noise that obstructs the clarity of the dialogue. Imagine if we could remove all that background clutter and focus solely on the character’s voice, creating studio-quality sound.
Here’s a subtractive fix for the audio. It’s now much clearer, making it easier to understand what the character is saying. This demonstrates the power of the subtractive model in removing background noise to deliver crystal-clear audio.
Here’s another audio sample. As you’ll notice, the audio is somewhat difficult to understand—some frequencies may be missing. This snippet comes from in-flight satellite communication, where neither the technology nor the speaker is set up for studio-quality sound.
Finally, here’s a reconstructive fix. Because the reconstructive technology was able to repair the missing higher frequencies, the voice is more normal and the audio is studio-quality. This is someone who could be speaking next to you, rather than playing through your headphones.
And how about a visual aid? Here’s a series of spectrograms. They are visual representations of a signal’s spectrum of frequencies as it varies with time. In the example below, you can literally see the different AI-powered technology at work. In the original version, the high frequencies are lost as a result of a bad microphone or a speaker too far away from the microphone. In the subtractive model, incorrect frequencies are deleted but not repaired. And in the final reconstructive model, the higher frequencies have been repaired without any other signals being lost.
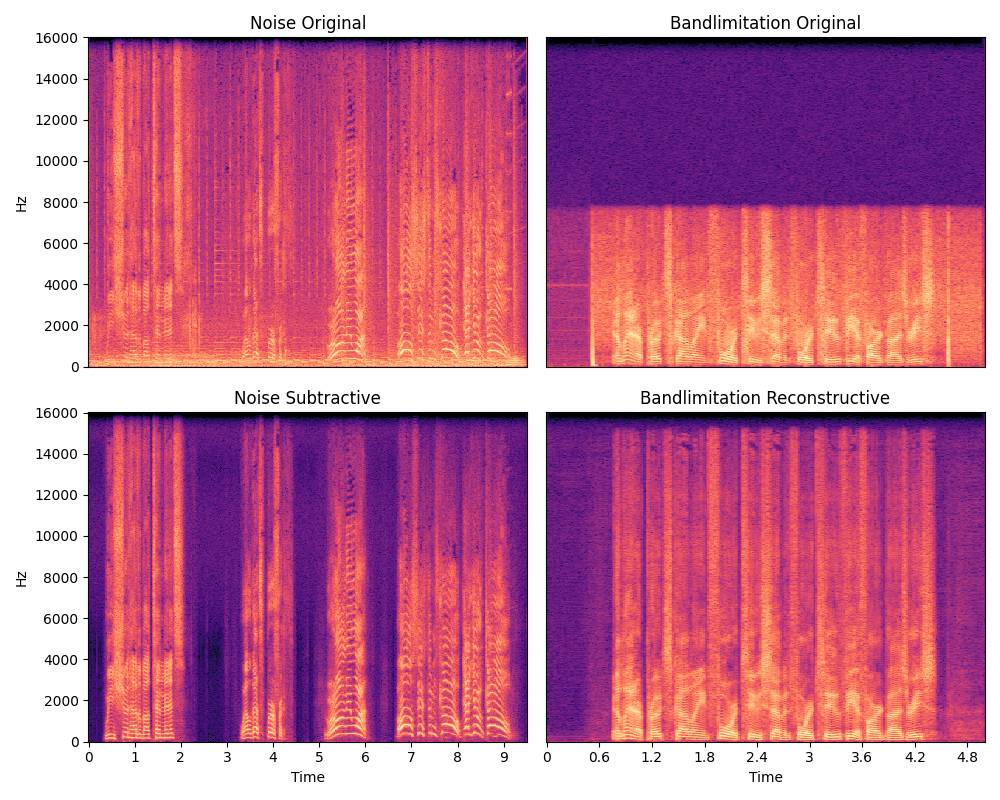
How does machine learning and AI-powered technology ethically reproduce someone’s voice?
It might sound worrying to have AI-powered technology replicating someone’s voice. But that’s not really what our reconstructive model is doing — instead, it’s repairing your or your subject’s voice. Often those missed signals are very minute, only nanoseconds long; rather than repairing a full sentence, it might be repairing a word, or a syllable, or even less than that! But it all adds up to clearer, more professional and understandable audio. And even when a longer repair is necessary, the technology is working off someone’s existing voice patterns to simply replicate the missing words.
That also means that our technology doesn’t ever change speaker identity. We do not clone voices, which means there’s no option to steal voices or create deep fakes with our technology. Unlike our voice cloning and text-to-speech cousins, ai|coustics never changes any specifics about how someone sounds or speaks. Instead, it preserves the speaker’s voice, timbre, intonations and accent.
Our technology can do this because of a machine learning model based on huge amounts of data training. Here at ai|coustics, we train the model on both high quality and degraded audio, so that the model can begin to answer questions like: Does this audio contain speech? Where is the speech? Does it contain noise? Do I need to add or substract information? Is the frequency unbalanced?
By teaching the technology exactly how high-quality audio sounds, it can recreate that sound, whatever the input.
Ready to try it for yourself?